The Rise of Generative AI: Developing Enterprise Solutions and Its Role in Mergers, Acquisitions, and Incident Response
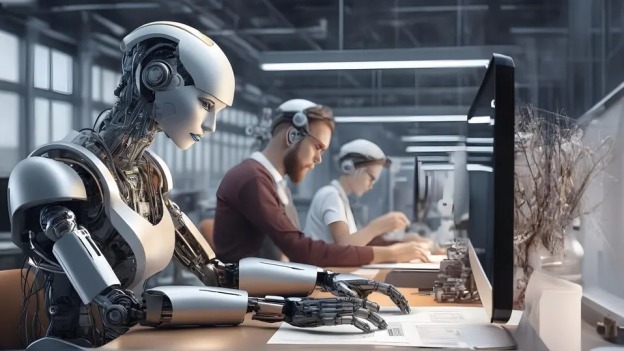
Generative AI is transforming how enterprises operate, innovate, and respond to challenges. From enhancing decision-making processes to streamlining complex operations, the applications of Generative AI are far-reaching. This article delves into how to develop Generative AI for enterprises and explores its significant roles in mergers and acquisitions (M&A) and incident response.
Developing Generative AI for Enterprises
Understanding Generative AI
What is Generative AI?
Generative AI refers to algorithms that can generate new data or content by learning from existing data. This includes creating text, images, audio, and even synthesizing information. Unlike traditional AI, which focuses on recognizing patterns, Generative AI can produce entirely new outputs.
Why Enterprises Need Generative AI
Enterprises can leverage Generative AI to automate content creation, enhance customer interactions, and streamline operations. This technology can reduce costs, improve efficiency, and foster innovation across various departments, making it a critical tool for modern businesses.
Steps to Develop Generative AI for Enterprises
1. Identifying Business Needs
The first step in developing Generative AI for enterprises is identifying specific business needs. This involves consulting with stakeholders to understand pain points, opportunities, and desired outcomes.
2. Data Collection and Preparation
Generative AI relies on vast amounts of data. Enterprises must collect and prepare high-quality data, ensuring it is clean, relevant, and structured. This data serves as the foundation for training AI models.
3. Choosing the Right Model
Selecting the appropriate AI model is crucial. Common models for Generative AI include Generative Adversarial Networks (GANs), Variational Autoencoders (VAEs), and transformer-based models like GPT-3. The choice depends on the specific application and data characteristics.
4. Training the AI Model
Training involves feeding the model with data and allowing it to learn patterns and relationships. This process requires significant computational power and expertise in machine learning to fine-tune the model and achieve optimal performance.
5. Validating and Testing
After training, the model must be validated and tested to ensure it meets business requirements. This involves running simulations, evaluating performance metrics, and making necessary adjustments.
6. Deployment and Integration
Once validated, the AI model can be deployed and integrated into existing systems. This requires collaboration between AI developers, IT teams, and business units to ensure seamless implementation and adoption.
7. Continuous Monitoring and Improvement
Generative AI models need continuous monitoring to maintain their effectiveness. Regular updates, retraining with new data, and performance assessments are essential to keep the AI solution relevant and efficient.
Enhancing Due Diligence
Automating Data Analysis
AI can automate the analysis of vast amounts of data during the due diligence process. This includes financial records, legal documents, and market research. By quickly identifying patterns and anomalies, AI reduces the time and effort required for thorough due diligence.
Identifying Synergies and Risks
AI can help identify potential synergies between merging companies, such as complementary products or services, and highlight risks, such as cultural mismatches or overlapping roles. This comprehensive analysis enables better decision-making and strategic planning.
Facilitating Integration
Streamlining Processes
Post-merger integration is often complex and challenging. AI for mergers and acquisitions (M&A) can streamline processes by automating repetitive tasks, coordinating between departments, and providing real-time insights. This ensures a smoother transition and quicker realization of merger benefits.
Cultural and Operational Alignment
AI tools can assess cultural compatibility and operational alignment between merging entities. By analyzing employee sentiment, communication patterns, and workflow efficiency, AI helps in designing strategies to harmonize different organizational cultures and operational practices.
Optimizing Valuation and Negotiation
Predictive Analytics
AI’s predictive analytics capabilities can forecast future performance, market trends, and potential risks. This information is invaluable during valuation and negotiation phases, enabling more accurate and data-driven decisions.
Scenario Planning
AI can simulate various scenarios and their potential outcomes, helping stakeholders understand the implications of different decisions. This facilitates better preparation and negotiation strategies, ultimately leading to more favorable terms.
AI in Incident Response
Enhancing Detection and Prevention
Real-Time Monitoring
AI systems can continuously monitor networks, systems, and applications for suspicious activities. By leveraging machine learning, AI can detect anomalies and potential threats in real time, allowing for quicker response and prevention of incidents.
Predictive Threat Analysis
AI in incident response can predict potential threats by analyzing historical data and identifying patterns associated with malicious activities. This proactive approach helps organizations anticipate and mitigate risks before they escalate into incidents.
Automating Response Actions
Immediate Containment
When an incident is detected, AI can automatically initiate containment measures, such as isolating affected systems, blocking malicious IP addresses, and restricting user access. This rapid response minimizes damage and prevents the spread of threats.
Incident Triage
AI can triage incidents by assessing their severity, impact, and urgency. By prioritizing incidents and assigning them to the appropriate response teams, AI ensures that critical issues are addressed promptly and efficiently.
Improving Recovery and Learning
Data Recovery
AI tools can assist in data recovery efforts by identifying and restoring compromised data from backups. This ensures business continuity and minimizes downtime following an incident.
Post-Incident Analysis
AI can analyze post-incident data to identify root causes, assess the effectiveness of response actions, and recommend improvements. This continuous learning process enhances the organization’s resilience and prepares it for future incidents.
Conclusion
Generative AI is revolutionizing how enterprises operate, innovate, and respond to challenges. From enhancing customer interactions to streamlining mergers and acquisitions and improving incident response, the applications of Generative AI are vast and impactful. By understanding how to develop and implement these solutions, businesses can harness the full potential of Generative AI to drive growth, efficiency, and resilience in an increasingly competitive landscape.
Embracing Generative AI is not just about adopting new technology; it’s about transforming business processes and strategies to meet the demands of the future. With continuous advancements and increasing adoption, the role of Generative AI in shaping the future of enterprises across various sectors, including customer service, payments, lending, and beyond, will only become more pronounced.