Smarter, Not Larger: How Small Language Models Are Transforming AI Strategy?
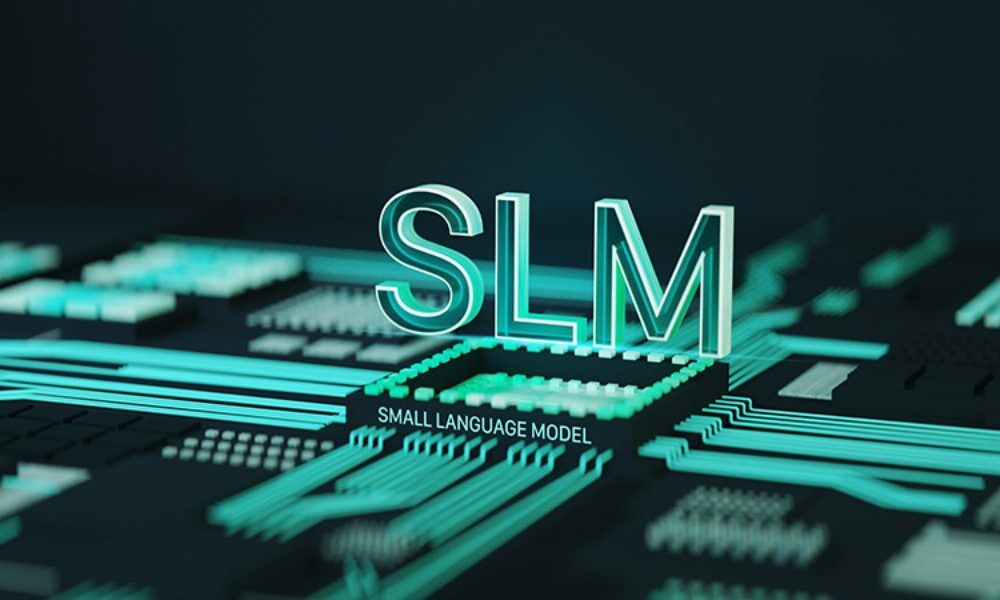
In the rapidly advancing world of Artificial Intelligence, the allure of extremely large models like OpenAI’s GPT-4, GPT-3.5 is undeniable. These models, with their billions of parameters, are designed to handle a vast array of tasks, offering immense computational power. For instance, GPT-3.5, with 175 billion parameters, has become a benchmark in AI for its ability to generate human-like text, solve complex problems, and even code. However, for many organizations, the cost of deploying such models can quickly escalate. According to recent estimates, running a single inference of GPT-4 on a commercial cloud platform can cost $120 for 1M tokens or 750k words approximately.. This might seem trivial for occasional tasks but becomes prohibitively expensive when scaled across millions of queries or when integrated into everyday operations.
In contrast, tasks such as summarization, Q&A chatbots, and document retrieval can often be effectively accomplished with Small Language Models (SLMs), which typically have a fraction of the parameters. For example, a model like Mistral 7B, with just 7 billion parameters, has demonstrated efficiency in performing these tasks with a reduction in costs by up to 90% compared to larger models. When optimized correctly, these smaller models provide a powerful and cost-effective alternative without significantly compromising performance. SLMs have been shown to reduce inference costs to as low as $.1.26 per 1M tokens , a hundredfold decrease, while maintaining up to 90% of the accuracy for specific tasks. This drastic reduction in cost, combined with near-parity in performance, makes SLMs not just a viable option but often the preferred choice for organizations aiming to maximize their return on investment in AI.
By focusing on practical applications where extreme model size is not a necessity, organizations can allocate resources more efficiently, ensuring that AI initiatives are both impactful and sustainable. Vivek, a Senior AI/ML Specialist Solutions Architect at AWS, is at the forefront of promoting SLMs as a practical solution for organizations seeking to balance performance and cost. By focusing on techniques like Retrieval-Augmented Generation (RAG), prompt engineering, and fine-tuning, Vivek demonstrates how these models can access the latest proprietary data, ensuring up-to-date and relevant responses while maintaining the efficiency and transparency that SLMs are known for. For instance, Vivek highlights the fine-tuning of Mistral 7B, a model that runs efficiently on a single A10G GPU, performing exceptionally well while saving costs by over 90% compared to larger LLMs that require much more powerful GPUs.
Why Choose Small Language Models? The Benefits and Optimizations Explained
In a landscape where extremely large models often dominate the headlines, Small Language Models (SLMs) are proving to be a compelling alternative for many organizations. But why should companies consider SLMs over their larger, more powerful counterparts?
Vivek offers a clear perspective: “SLMs democratize access to AI by being more accessible to organizations of all sizes, regardless of budget or affiliation. Their transparency allows for greater control, enabling companies to understand exactly how the models operate and effectively mitigate potential biases or errors.” One of the standout advantages of self hosting SLMs is their ability to maintain privacy and data security. As Vivek points out, “With SLMs, there’s no need to send sensitive data to black-box APIs. This means organizations retain full control over their data and can safeguard their intellectual property while training and owning models on proprietary datasets.”
The flexibility of SLMs further enhances their appeal. “SLMs offer freedom of choice,” says Vivek. “Organizations aren’t locked into a single provider, and they can switch models or platforms as needed, deploying them on any technology stack they prefer.” This IT flexibility is crucial for companies looking to integrate AI seamlessly into their existing infrastructure. Cost optimization is another significant factor driving the adoption of SLMs. “Finding the right cost/performance balance is key,” Vivek explains. “Techniques like prompt engineering, quantization, speculative decoding, and batching allow us to fine-tune SLMs for specific tasks, ensuring high performance while staying within budget constraints.”
Vivek’s work with SLMs underscores their practical benefits: accessibility, transparency, privacy, flexibility, and cost-efficiency. These factors make SLMs an attractive option for organizations aiming to optimize their AI strategies without compromising on quality or control.
How Can SLMs Deliver High Performance?
In the world of AI, where bigger often seems better, how can Small Language Models (SLMs) deliver high performance? The answer lies in the strategic optimizations championed by experts like Vivek Gangasani. “SLMs can achieve remarkable results without the heavy overhead associated with larger models,” says Vivek. “The key is in the fine-tuning process, where we customize these models to the specific needs of a business. In many cases, a small, well-tuned model can outperform a larger, vanilla model, especially when the task is well-defined.”
One of the techniques Vivek emphasizes is prompt engineering, which refines how tasks are framed and inputs are structured. “By carefully crafting prompts, we can significantly enhance a model’s output quality,” Vivek explains. “When paired with Retrieval-Augmented Generation (RAG), which allows models to access and incorporate the latest data, SLMs can deliver high-quality results in real-time applications.”
The strategic combination of these optimizations with the inherent advantages of SLMs—such as accessibility, transparency, privacy, and cost-efficiency—creates a powerful AI solution. “Choosing the right model for the task at hand is crucial,” Vivek advises. “It’s not always about selecting the biggest or most expensive option but about finding the model that best fits the job.”
Through his work, Vivek has demonstrated that with the right frameworks and libraries, SLMs can boost performance by up to 60%, achieving enhanced throughput while maintaining cost-effectiveness. This approach not only maximizes efficiency but also ensures that organizations remain agile and competitive in the rapidly evolving AI landscape.
What Does the Future Hold for AI? The Growing Role of Small Language Models
As the field of AI continues to evolve, what does the future hold for organizations seeking to balance innovation with cost-efficiency? According to Vivek, the answer lies in the growing importance of customizable, cost-effective models like Small Language Models (SLMs). “The landscape of AI is shifting,” Vivek notes. “As businesses become more data-driven, the demand for models that are both powerful and affordable will only increase. SLMs are uniquely positioned to meet this demand, providing organizations with the flexibility to tailor solutions to their specific needs without breaking the bank.”
Vivek’s pioneering work with SLMs showcases their potential, offering both technological and economic advantages that are becoming increasingly hard to ignore. “We’ve seen firsthand how SLMs can deliver on performance while keeping costs in check,” he says. “This makes them an ideal choice for companies that want to stay at the forefront of AI innovation without the financial burden of maintaining larger models.” Looking ahead, Vivek emphasizes the strategic importance of adopting SLMs as part of a broader AI strategy. “Organizations that embrace SLMs will not only optimize their AI operations but also position themselves as leaders in a rapidly changing field. The key is to remain agile, adapting to new technologies while keeping an eye on cost-effectiveness.”
Conclusion
As we’ve explored, Small Language Models (SLMs) are emerging as a game-changing force in the AI landscape. Their ability to deliver high performance at a fraction of the cost of larger models is revolutionizing how organizations approach AI implementation. By offering accessibility, transparency, privacy, and cost-efficiency, SLMs are democratizing AI technology, making it available to businesses of all sizes. The insights provided by experts like Vivek Gangasani highlight the transformative potential of these models when coupled with strategic optimizations such as fine-tuning, prompt engineering, and Retrieval-Augmented Generation. As AI continues to evolve, the role of SLMs is set to grow, offering a balanced approach that combines innovation with practicality.
For organizations looking to stay competitive in the AI race without compromising on budget or control, Small Language Models represent not just an alternative, but potentially the future of sustainable and efficient AI strategy. As we move forward, the ability to leverage these nimble, powerful tools may well become a defining factor in an organization’s success in the AI-driven world.